It’s Early for Artificial Intelligence in ICS Cybersecurity
AI may have significant potential for ICS cybersecurity, but at present, industrial companies looking to deploy the technology should proceed with caution.
March 14, 2019
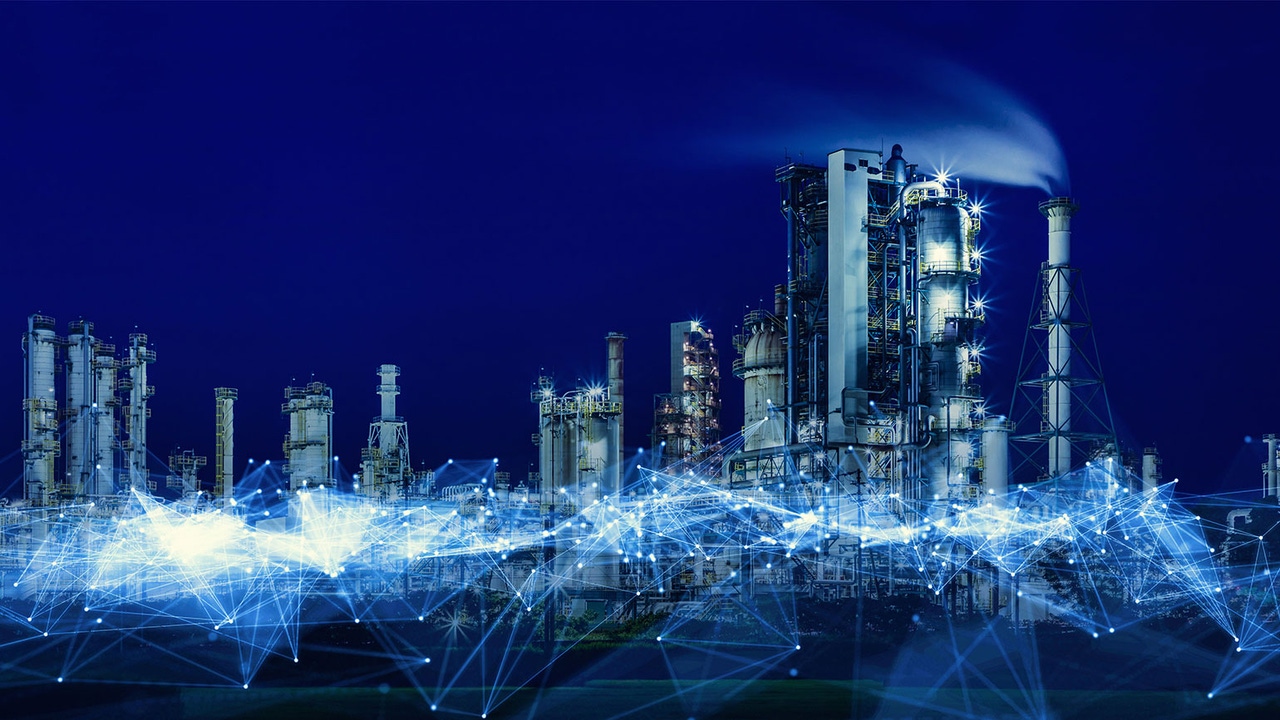
A virtual assistant developed by Google made waves when it debuted last year because it sounded indistinguishable from a human when phoning a restaurant to make a reservation. “Hi, I’d like to reserve a table for Wednesday, the seventh,” beamed the polite-sounding male voice in the Google Duplex demo. “For seven people?” asked a woman on the other end of the line, apparently misunderstanding. “It’s for four people,” retorted the virtual assistant, prefacing that statement with a natural-sounding “um.”
The example of Google Duplex serves as a microcosm for the current state of AI. Now available in 43 U.S. states for Google Pixel phone users, the Duplex system is at once impressive, but also calls to mind technological limitations. While Duplex may sound eerily like a human, its skillset is rather limited to rather routine interactions. By contrast, IBM’s Project Debater is more fluent in the abstract. It can give skilled human debaters a run for their money in terms of formulating arguments, but it presents its case in a flat robotic-sounding voice. Both the Duplex and Project Debater examples also call to mind the regularity with which the most-successful AI is the product of behemoth tech companies with massive budgets and data sets with armies of employees. And even then, top-tier companies are warning of the technology to potentially misfire. “AI algorithms may be flawed,” reads part of a recent Microsoft regulatory filing. “Datasets may be insufficient or contain biased information. Inappropriate or controversial data practices […] could impair the acceptance of AI solutions.”
[Internet of Things World is where industrial enterprises find IoT innovation. Book your conference pass and save $350, get a free expo pass, or see the IIoT speakers at the event.]
The generic marketing pitch for AI, however, is that the technology is a potential panacea for modern business problems — capable of helping enterprise and industrial companies make sense of mountains of data (including from IIoT devices) while also helping them boost the security of industrial control systems. “Industrial analytics, applied to machine data for operational insights, is as an engine driving the convergence of OT and IT, and ultimately value creation for the Fourth Industrial Revolution,” reads part of the intro from the Industrial Internet of Things Analytics Framework from the Industrial Internet Consortium.
When asked about the potential of AI for ICS cybersecurity, cybersecurity expert Jason Haward-Grau, CISO for PAS Global said “robotic process automation is probably far more interesting, from an AI perspective, than AI is in security,” referring to the business process automation technology that can reduce the need for human involvement in tasks such as procurement.
Yet the vendor landscape is thick with companies who have an AI offering for nearly any imaginable problem. “If you asked anybody: ‘Have you got AI?’ they’ll always say ‘yes.’” Haward-Grau said. “But define what it is. Ask the question: ‘If AI is the answer, what is the question?’ Because you are better off starting asking: ‘What does my business need?’”
The threat level is significant in ICS cybersecurity. A total of 49 percent of 321 industrial respondents suffered at least one attack annually, according to 2018 Kaspersky research. The actual figure could be higher, Haward-Grau said, because the aforementioned figure represents attacks organizations are willing to admit have happened.
At present, the term AI is used in a myriad of ways and definitions of the term can seem philosophical because it remains difficult to understand in concrete terms what intelligence is. “From an engineering point of view, it is difficult to define ‘smart,’” said technology writer Jaron Lanier in a 2016 debate on AI. “If you don’t define a baseline that is measurable, you are off in fantasy land.” He also added that: “A lot of the systems we call ‘smart’ systems are kind of derailed from the empirical process.”
One proposed use case for AI systems, or to be more precise, machine learning, is its use for detecting malware or anomalies on a network. If you have a baseline of how the network should operate and have sound machine learning algorithms and sufficient data access, the technology can be powerful in quickly detecting network threats and, over time, potentially reducing the number of false alarms for potentially suspicious code or network behavior. Given the fact that the broader cybersecurity industry is wrestling with a considerable shortage of talented workers, that’s a big promise.
But in order for it to succeed, the machine learning system needs to have access to relevant data. If the business is doing something that the AI system isn’t aware of, you can have problems — in the form of false alarms. Or perhaps the supervised learning system designed to investigate software code was trained on bad data, leading the algorithm to potentially deem malware as normal. In addition, adversaries could also modify a security vendor’s software to pass off malware as normal code. Another possibility, mentioned in a Technology Review article, is that attackers simply figure out the features the machine learning model is using to identify malware, and remove them from their own malicious code.
In an industrial context, it can be difficult to weave in data from equipment that is not IT-network-oriented or that don’t use the IT TCP/IP protocol. “How does AI work on a 25-year-old control bus?” asked Haward-Grau.
To provide an example of the potential difficulty in launching a wide-scale IoT project in an industrial environment, Haward-Grau gives the example of a refinery, which has 500 traditional IT devices such as physical workstations, HMIs, servers and switches. “It’s manageable. It’s like a small office. I could put network tracking around it,” he said. But then when the head of security asks the refinery how many OT endpoints it has, the answer is 28,500. While one of the advantages of AI, in general, is its potential to make sense of massive volumes of varied data, generated at a brisk velocity, in reality, it is still challenging to make sense of complex, historically siloed data. “The challenge isn’t the number” of endpoints, Haward-Grau said. “It is the challenge of having 20 different vendors. Let’s say I have equipment from ABB, Schneider Electric, Siemens, Yokogawa, Philips, GE and Honeywell,” he said. “They’re all different, they will talk differently. So how are you going to translate all those different things for a start and then answer the question: ‘What does good look like?’” Haward-Grau asked.
Add to that the shift in stance in cybersecurity from the assumption that it’s only a matter of time before companies get breached to the assumption that your firm has already been breached, the complexity of understanding what good network behavior looks like becomes more daunting. A 2018 study backed by IBM found that it takes enterprise companies an average of 197 days to identify a breach. That is bad news for organizations that are potentially compromised that are looking to train machine learning models on complex networking topologies.
All this is not to say that AI doesn’t have considerable potential for ICS cybersecurity, it’s just that the industrial companies looking to deploy the technology should start with defined use cases with initially limited data complexity. As the E. F. Schumacher once wrote, “Any intelligent fool can make things bigger, more complex, and more violent. It takes a touch of genius — and a lot of courage to move in the opposite direction.”
About the Author(s)
You May Also Like