Microsoft’s AI Model Identifies When Medical Treatments Harm More Than Help
Dead-end Discovery uses the reinforcement learning ML framework because it's well-suited for health care
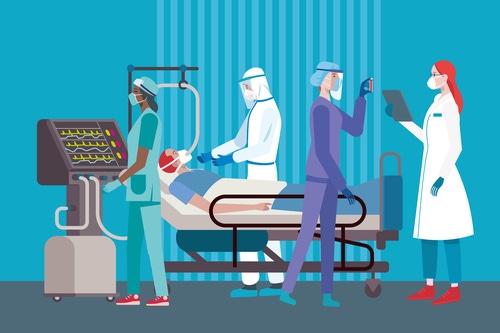
Microsoft researchers have built an AI model that can identify when medical treatments meant to help patients actually ended up harming them.
The model, Dead-end Discovery, uses the reinforcement learning ML framework – where an agent learns from trial and error – because it is well-suited to health care. Health care is characterized by sequential decision-making: After looking at a patient’s condition, providers apply a treatment and observe the results. If the patient improves, the process repeats.
Medical care today is characterized by a focus on what to do to help the patient recover. But the researchers argued that this can be an “infeasible” goal. Instead, they propose an opposite approach: identify treatments to avoid and prevent a point of no return for the patient.
The researchers applied their model on real-world medical data that was publicly available, focusing on critically ill patients with sepsis in intensive care.
Their goal was to help providers “identify which subset of treatments could statistically cause further health deterioration so they could eliminate them when deciding on the next steps,” the researchers said in a blog.
They used a dataset of 53,400 hospital admissions between 2001 and 2012 and extracted a group of nearly 20,000 ICU patients with sepsis. (They applied offline reinforcement learning because they used a fixed dataset.) The researchers studied 72 hours of the patients’ stay in ICU, 44 observation variables and 25 treatments. They also used their AI model to identify patients showing signs of slipping into death.
The results: More than 12% of treatments given to patients who later died could have been detrimental – 24 hours before they passed away. Also, the AI model identified as much as 10 percent of patients slipping into a point of no return up to 48 hours before they died.
“While these percentages may seem small, more than 200,000 patients die of sepsis every year in U.S. hospitals alone, and any reduction of this rate would result in possibly tens of thousands of individuals who would otherwise survive,” they said.
The researchers said further uses of their AI model could include other areas of health care. Another possible application could be in finance, to alert investors when certain buy or sell decisions are likely to lose money – a financial dead end.
To access the code: https://github.com/microsoft/med-deadend
This article first appeared in IoT World Today’s sister publication AI Business.
About the Author(s)
You May Also Like