AI Frameworks Reduces Generative AI’s Risks, Applied Intelligence Live! Austin 2023
American Airlines senior data scientists Sai Nikhilesh Kasturi says an ethical framework will accelerate adoption of generative AI
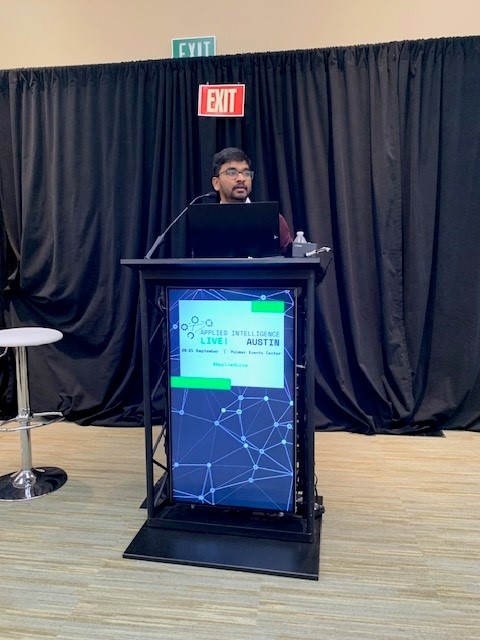
The tipping point that ushered in the era of generative AI came from the confluence of three factors: massive proliferation of data, advances in scalable computing and machine learning innovation.
But as awe-inspiring as the capabilities of generative AI are − be it text-to-image, text-to-text, text-to-video and others – adoption is being hindered by its well-known risks including bias, privacy issues, IP infringement, misinformation and potential toxic content.
“These are the high-level risks and concerns that every company or organization sees right now, and that’s the whole reason they are a little skeptical of using ChatGPT for their daily work,” said Sai Nikhilesh Kasturi, a senior data scientist at American Airlines at Applied Intelligence Live! in Austin, Texas.
His solution to mitigate these risks? Establish the right AI frameworks.
Strategy and control
AI policy and regulation
Governance and compliance
Risk management
Responsible practices
Model interpretation
Transparent model decision-making
Bias and fairness
Define and measure fairness
Test
Security and safety
Core practices to fine-tune model output
Follow industry standards and practices
Keep humans in the loop
Monitor against model drift
“Once the ethical frameworks are built, and they are in place, the massive adoption of generative AI might increase over the years,” Kasturi said, citing Bloomberg’s prediction of market growth to $1.3 billion by 2032.
Traditional AI models were used for one specific task but the foundation models underpinning generative AI are being used for various tasks at the same time such that the training time has been reduced “drastically.”
Asked how one can solve a sticky problem in generative AI of getting answers wrong – or making them up – Kasturi said one way is to use two AI systems to cross-check each other.
MIT and Google DeepMind researchers recently developed a method in which AI chatbots get to the right answer by debating each other.
This article first appeared in IoT World Today's sister publication AI Business.
Read more about:
Applied Intelligence Live!About the Author(s)
You May Also Like