Machine Learning Is Essential for IoT Security, But Can't Do It Alone
Machine learning has a critical place in protecting our future IoT devices but cannot answer for every security challenge.
June 7, 2017
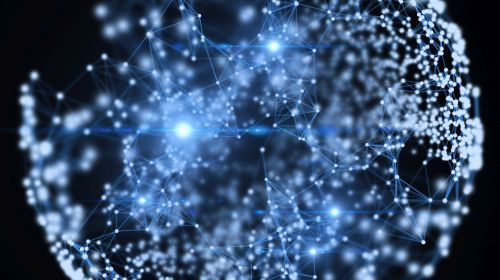
IoT and connected devices are appearing in both homes and businesses at breakneck speed, and we now need solutions able to detect cyberattacks quickly and efficiently without wasting human resources.
Machine learning will be key, security experts say, but cannot be the Holy Grail of IoT security. Instead flexible solutions leveraging human security expertise, deep learning and artificial intelligence are the only way forward.
IoT encompasses everything from household products to mobile devices which are connected to networks, whether locally or via the web. Everything from a smart fridge to monitoring a fleet of corporate cars and recording data on energy usage is possible through connected devices, but also increases the burden on security professionals to keep corporate networks and resources secure, thanks to a rapidly increasing attack surface.
According to Hal Lonas, chief technology officer at cybersecurity firm Webroot, machine learning and artificial intelligence can help cybersecurity experts take on this challenge, especially as the problem is made more complex due to many IoT vendors considering security little more than an afterthought.
“The security industry needs to get ahead of the problem we’re creating, and the only way to do that is with automation,” Lonas says.
IoT is a new and immature market. Outdated firmware, zero-day vulnerabilities and poor patching cycles are only the tip of the threat iceberg, and as threat actors become more sophisticated, so will attack vectors.
The emergence of virtual reality (VR) adds to the burden. VR and augmented reality (AR) technology is now appearing in headsets, mobile apps and games, collecting even more data from us than standard e-commerce companies, tracking cookies and social media.
While some of this data is collected by the likes of Oculus and Microsoft and is beneficial to vendors and advertisers, this treasure trove of deeply personal data also must be kept safe from intrusion.
Should only one vulnerable IoT, mobile or gaming device slip through the net, an entire network and the personal data of users stored within could become a cyberattacker’s playpen.
Perhaps, one day, machine learning could prevent such breaches occurring.
“Machine learning is not a silver bullet, but it is an essential tool in the box for keeping ahead of, or at least quickly detecting, some of the latest types of attack,” Lonas says.
Machine learning is nothing new. The concept first emerged in the 1900s and has expanded to include deep learning, data analysis, pattern detection and, for the purpose of security, anomaly alerts.
Analysts set with the task of monitoring connected devices on a corporate network have only so much time and so many tools at hand to watch over all network entry points and potential avenues for exploit.
However, machine learning can provide the bridge to utilize the vast amounts of data that IoT devices produce and compile this information into patterns which can be used to understand whether a device or not is compromised — as well as alert operators when suspicious activity is detected.
“Machine learning can monitor thousands of variables, versus the handful that people can, all while never getting tired, lazy, or taking a day off,” Lonas said. “This in turn frees up analysts’ time to work on more valuable areas of security, reducing the time between a threat being introduced and our ability defend against it.”
Machine learning can also limit human error and reduce costs, but the technology is still within early developmental stages and there are many hurdles which need to be overcome before such solutions will be viable for IoT security.
Lonas says it is “incredibly hard to get machine learning right” due to the need to balance algorithms with performance, as well as deal with the requirement to compile threat alerts into publishable notices on time. Merging AI and advanced technology with legacy systems would also, likely, prove a challenge for businesses — assuming they wish to invest in machine learning security in the first place.
Today analysts can only digest less than 10% of the unstructured security data available, Caleb Barlow, vice president of IBM Security, told IoT World News. If vendors and enterprise players are going to utilize data flows effectively to keep products and networks safe from cyberattack, machine learning is a requirement to leverage data which is currently wasted.
Machine learning cannot, however, be the one-stop solution to IoT security. False positive and false negatives will crop up, and this is when human operators must come into play. Machines are good at repetitive tasks, but humans are the best option for applying technology to problem solving.
AI solutions may be able to utilise deep learning to deal with small attacks without assistance, but human operators will also still need to teach AI how to cope with more sophisticated attacks going forward.
There needs to be a “sweet spot”, says Lonas, between unsupervised and supervised machine learning “in order to both deflect attacks and uncover new vulnerabilities”.
“Fewer people needed for greater accuracy and coverage is the goal, but this will never get to zero,” Lonas continued. “The bad guys will continue to invent new attacks, so people will always be needed to innovate our defences.”
According to Simon Crosby, chief technology officer at Bromium, the adoption of machine learning is likely to be difficult due to a small number of people skilled in AI, the implementation of domain-specific languages for machine learning solutions, overall investment and the shift of traditional security teams to automatic workflows.
Vendors need to work on minimizing these problems if machine learning is going to take a prominent place in IoT security, not only in the enterprise but in the VR realm and consumer domain, including gaming and mobile devices.
“The industry is still in its infancy,” Crosby added. “The big providers — Apple, Google, Microsoft — already apply machine learning to the consumer world. The opportunity remains for other vendors to apply machine learning techniques to solve enterprise problems, and that market is nascent at best.”
About the Author
You May Also Like