Predictions for Embedded Machine Learning for IoT in 2021
Experts predict that embedded ML will make strides in 2021, as microcontrollers become more advanced and TinyML takes hold at the edge.
December 10, 2020
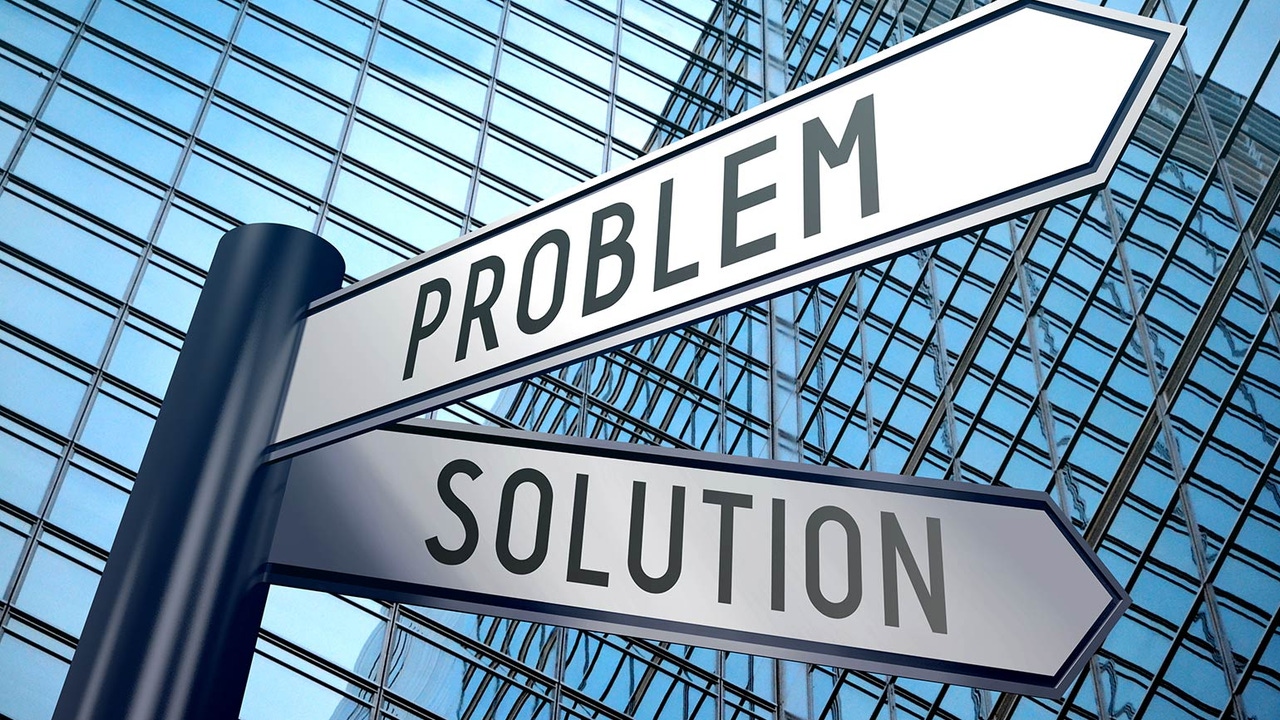
By Evan Schuman and Lauren Horwitz
Key takeaways from this article are the following:
From increasingly capable hardware to TinyML, embedded machine learning will make strides in 2021.
More capable microcontrollers combined with on-device machine learning at the edge is poised to develop further in 2021. These developments with further advances in video surveillance, manufacturing and more.
The impact of COVID-19 on the global supply chain, however, may stunt innovation and growth of embedded machine learning.
Despite silicon shortages, several new capabilities for embedded machine learning on Internet of Things devices will emerge in 2021, industry watchers predict.
New capabilities mean severing the cord between so many Internet of Things ( IoT) devices and the cloud and instead running processes at the edge. The boost in chip processing capabilities—which will continue to increase next year, as Moore’s Law dictates—means sidestepping cloud-based latency issues, among other benefits.
Experts argue that moving processing to the edge – or “going to local execution,” as Hiroshu Doyu,, an embedded AI researcher at Ericsson, puts it –will deliver five distinct advantages in 2021:
Network bandwidth
Network coverage
Latency
Power consumption
Privacy
Privacy will be less “porous,” Doyu said, offering fewer opportunities for data to be stolen while in transit to the cloud or on the return trip. “Once the AI is more powerful, that kind of device can be installed without a power line,” he said.
“More powerful IoT AI chips will be shipped and more domain-specific IoT AI chips will be shipped. Both would enable smarter intelligence on IoT sensors,” Doyu said.
TinyML Fueled by More Capable Hardware, Dev Trends
Lest we undervalue the significance of this, it’s important to note the dynamics underlying more intelligent hardware. It is the result of the convergence of several trends.
First, in recent years, hardware advancements have enabled the microcontrollers that perform calculations much faster. Improved hardware combined with more efficient development practices have made it easier for developers to build programs on these devices. And third, is the rise of tiny machine learning, or TinyML, has ushered in this era of smarter hardware.
TinyML encompasses technologies capable of performing on-device analytics garnered from sensor data at low power. Together with hardware advancement and progress in ML development, microcontrollers can now run increasingly complex ML models directly on the hardware and without a round trip to the cloud.
“The current dumb IoT sensors would become smarter with TinyML.” Doyu said.
To further expand TinyML on device, many enterprises will turn to TinyML as a service (TinyMLaaS)., in which an IoT device concretely takes part in the execution of intelligent services. It’s difficult to find competency in both embedded and machine learning at the same time. But for the next wave of IoT sensors, TinyML must be installed by IT pros who understand embedded and ML, where TinyMLaaS does the work for them, Doyu said.
Proliferation of Smarter Chips Will Force Enterprise Maturation
“The adoption of way more intelligence at the device level bypasses a lot of the issues, especially with bandwidth and latency,” said Lucy Lee, a senior associate at Volition Capital who tracks embedded AI/ML on IoT. She also predicts many more autonomous chips a year from now.
But Lee stressed that much of this relies on enterprises cleaning up existing systems. Enterprise IT execs are “completely inundated with the tech stuff we have already embedded. The problem is connecting to it and ingesting it,” Lee said, offering an example in the transportation industry.
“Railway operations — they don’t have the capacity to care about predicting downtime when they currently can’t even track which motors are on or off,” Lee said.
Lee also cited autonomous vehicles as an area where she said that she expects a lot of improvements, mostly via disconnecting from the cloud. She detailed some work with embedded cameras designed to detect oncoming traffic. Once insights can be delivered without the delays inherent in cloud communications, lives can be saved, she said.
“By using the edge instead of the cloud, those extra 100 or 50 milliseconds that are saved do become life saving and mission critical,” Lee said, adding that cloud operations cannot always be reached instantly.
Lee said that enterprises’ current overpopulation of IoT devices The thousands of devices already installed will be eclipsed by the next generation of smarter hardware.
“What [customers] want are smarter ways for that data to be ingested, analyzed and insights to be generated,” Lee said. “A lot of IoT [devices] are coming in with really clever software platforms that can plug and play with a wider range of devices to either breathe life into existing infrastructure and extract value out of them, or displace some existing infrastructure through consolidation to lighten the IT stack that industrial customers need to maintain.”
Computer vision is an IoT area where Daniel Elman, a Nucleus Research analyst, expects critical improvements. It will involve training data sets with few-shot and one-shot learning, which involves training models with less data than is traditionally required.
“Few-shot and one-shot learning, an approach to deep learning that allows models to be accurately trained on very small data sets, has seen considerable progress this year and has the potential for practical IoT use in 2021,” Elman said.
“Few-shot and one-shot learning allows you to train a system to identify numbers, but rather than feed it thousands of differently-presented numbers, you would only feed it the digits 0-9, and for each digit, ascribe a percentage for how much it looks like the others.”
Elman is referring to the visual depiction of the shape of the numbers, rather than what they mathematically represent. “For example, the digit 3 is half of the digit 8 and about a third the same as the digit 5,” Elman said. Because “there isn’t a one-to-one relationship between the training data point and its output, this dramatically reduces the amount of data needed to train the model. This type of learning could significantly benefit edge/IoT devices,” Elman said.
For smaller devices with limited memory and compute, it’s not feasible to run or train full-scale deep learning models that often have billions of mathematical operations. With this new approach, the models can be compressed to run more efficiently on these IoT devices.
On a practical level, Elman said, this might allow for a security camera to decide on its own whom to allow into a secure room and whom to lock out. Elman cited some current work at Massachusetts Institute of Technology that could easily be commercialized by 2021.
Embedded ML Could Hit Speed Bump Given COVID-19 Fallout
Elman also noted progress in manufacturing environments.
“Forecasting is at the center of so many ML/AI conversations this year as companies look to leverage deep learning and neural networks to forecast time series data,” he said. The value … is the ability to ingest data and immediately realize insight into how it changes the long-term outlook. Think of a receiver at a factory waiting for a delivery. If a shipment shows up with half the components it was supposed to, being able to understand clearly how this will affect the financial and production outcomes into the future is certainly a critical priority.” Elman noted, however, that it’s still early days for these kinds of applications to IoT.
Further, much of the timing depends on the global supply chain, which makes developments much more difficult to accurately project.
“The pandemic has certainly slowed down the silicon supply chain, as the bulk of global production is based in the APAC region, specifically China and Taiwan, which have been hit particularly hard by the pandemic,” Elman said.
Lead times for various products have been affected, which reduces supply and can increase prices. Over the longer term, supply chain delays can even place a chokehold on innovation, Elman said “It could slow the development/large-scale adoption of these technologies as the resources need to be used in more established/essential technologies like cell phones, computers, and medical devices.”
You May Also Like