Diversity in Silicon Powers AI Innovation at the Edge
AI processing at the edge has spurred innovation among silicon vendors to enable these capabilities, with no vendor likely to dominate the market.
April 21, 2020
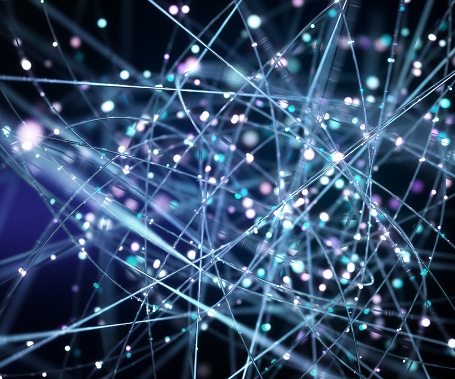
Key Takeaways:
A burgeoning silicon industry is developing intelligent chips to enable artificial intelligence at the edge and at the device level.
AI-enabled chips will enhance capabilities such as image recognition self-driving cars and voice-activated commands and more.
The influx of diverse chips may pave the way for new, unexpected uses of AI at the edge.
Intel dominated the CPU market for PCs, then servers. Most smartphones and tablets were powered by RISC-based chip technology licensed from Arm Limited. But a more diverse group of semiconductor providers is poised to accelerate artificial intelligence (AI) processing at the edge.
Innovation is blossoming amid a silicon renaissance as growing demand for AI processing capabilities fuels investment in logic processing chips for network edge applications. The diverse nature of Internet of Things (IoT) devices that will connect at the edge makes it less likely we’ll see this new frontier dominated as in the past by one or two chip makers.
“These edge AI chips will likely find their way into an increasing number of consumer devices, such as high-end smartphones, tablets, smart speakers and wearables,” Deloitte analysts reported. “They will also be used in multiple enterprise markets: robots, cameras, sensors and other [IoT] devices in general.”
AI chips feature parallel processing capabilities to execute a much larger number of calculations than traditional central processing units (CPUs) that execute instructions in a linear fashion.
“They also calculate numbers with low precision in a way that successfully implements AI algorithms but reduces the number of transistors,” according to the Center for Security and Emerging Technology. The ability of an AI chip to store an entire algorithm accelerates memory access while specialized programming languages running on the silicon efficiently translates AI code.
Next Generation of Deep Learning
Deep learning, a type of machine learning that was once the province only of supercomputers, has moved downstream to general enterprise systems that can readily recognize patterns among large streams of data. Essentially, this capability enables systems to make decisions from new data, based on what they previously learned from other data. The new generation of silicon components will bring deep learning down to the device level.
Most AI applications depend mainly on graphics processing units (GPUs) running in tandem with traditional CPUs on expensive, power-hungry data center servers, or on downsized servers located near the data source. Those implementations excel at training algorithms by analyzing large, historical data sets.
Enterprises and vendors are eager to deploy those trained algorithms in stand-alone inference models that operate at the device level. These models enable devices to automatically act on new information without requiring intervention from a remote server. That’s critical for devices that need to interpret sounds, images and other inputs in near real time. In an autonomous automobile, for example, they could distinguish a stop sign from a lamppost or bystander, or in a factory setting, halt a production line when a manufacturing equipment component appears to be misaligned or faulty.
Deep learning chips that can process data at the edge could propel a wave of applications, while also constraining the amount of remote data processing. Camera event recorders, for instance, already are in use with GPUs that integrate machine vision for enhancing risk detection — such as distracted driving — and can discern between data that needs to be uploaded to cloud servers immediately or used in the vehicle.
Many edge devices use low or intermittent power, lack heat dispersion capabilities and can operate dependably regardless of environmental factors such as temperature and movement. The chips that process instructions and images for servers, PCs and even today’s smartphones can’t meet those requirements and are generally too costly for many edge AI applications.
Pursuing Blank-Slate Approaches
GPUs are the workhorses of today’s AI applications, particularly in data center and cloud environments where they are increasingly employed to “train” AI algorithms that can develop inference models to be run at the edge. These chips are also finding their place in higher-value devices such as smartphones, automobiles and high-end appliances, or sophisticated industrial products such as factory-floor robotics systems.
Priced from tens of dollars to hundreds of dollars per unit, GPUs are not economically feasible for mass market, low-cost devices such as toasters, or in industrial applications such as electrical grid management systems that employ hundreds of thousands of microcontrollers in relays and switches.
New semiconductor providers are responding to demand for chip-embedded AI accelerator technology that can interpret and act on data without the latency and connectivity issues inherent in having to communicate with remote servers. The market for deep learning chipsets was forecasted to grow from $5.1 billion in 2018 to $72.6 billion in 2025, with edge computing devices accounting for 75% of the total market opportunity, according to the 2019 Omdia research report “Deep Learning Chipsets.”
“We need blank-slate approaches to process new types of AI models that utilize deep learning or its future enhancements,” said Aditya Kaul, research director with market research firm Omdia|Tractica and co-author of the deep learning chipset report. “We need to rethink processing architectures for doing matrix multiplications that currently dominate AI processing.”
Emerging AI Options
Dozens of emerging companies are pursuing alternate chip technologies that can accelerate AI at the edge in a more cost-effective manner than GPUs. Most of the startup activity is centered around application-specific integrated circuits (ASICs), according to Kaul. Device and component developers can work with chip foundries to manufacture custom ASICs for discrete purposes.
The growing fervor for AI applications is spurring investment in dozens of startups, as well as well-established players. It’s also getting increased attention from Google, which has developed its own ASIC, the Edge (TensorFlow Processing Unit) TPU for local inference processing. That chip extends Google’s TensorFlow open source machine learning platform to remote, low-power devices.
ASICs enable high-performance processing with low-power consumption, making them desirable for edge devices in many applications for which GPUs are overpriced or ill-suited. As the name implies, ASICs are optimized for a specific application, while GPUs are capable of more varied processing operations. Field-programmable gate arrays (FPGAs) sit somewhere in the middle, providing device manufacturers with close to ASIC-type performance, but a somewhat higher price point, in a chip that can be reprogrammed to serve changing needs.
ASICs and FGPAs can provide high-performance acceleration of AI processing in a compact, low-energy form factor that lends itself to a range of IoT devices and sensors. Furthermore, they can deliver AI functionality at a lower price point. According to the Deloitte report, “In volumes of thousands or millions, these chips will likely cost device manufacturers much less to buy: some as little as US$1 (or possibly even less), some in the tens of dollars.”
AI capabilities can also be incorporated into System on a Chip (SoC) semiconductors that incorporate multiple components such as a ASICs, GPUs or FGPAs along with a CPU, internal memory and input/output ports all in one chip, which are already widely used in midrange and high-end smartphones.
Battle for Supremacy
Unlike the early days of the PC industry, where the x86 architecture became a de facto standard for a hardware device, AI edge solutions represent a much more fragmented universe encompassing a diverse hardware landscape.
“For IoT and IIoT, there really isn’t one AI architecture yet that people have gravitated to,” said Richard Wawrzyniak, senior market analyst, ASIC & SoC, at Semico Research Corp. “The door is open for even very small companies to get it right and evolve and emerge to be major players.”
The diversity of chip options provides greater opportunity for non-semiconductor companies to apply technology to new uses. As device manufacturers, integrators and enterprises look to exploit AI at the edge, the silicon renaissance is poised to spur more innovation that can affect virtually any aspect of business and society.
About the Author
You May Also Like